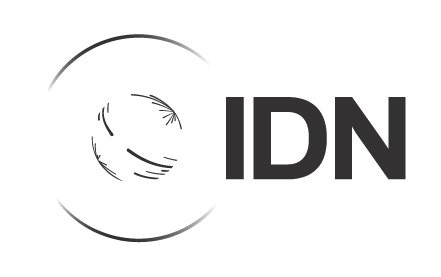
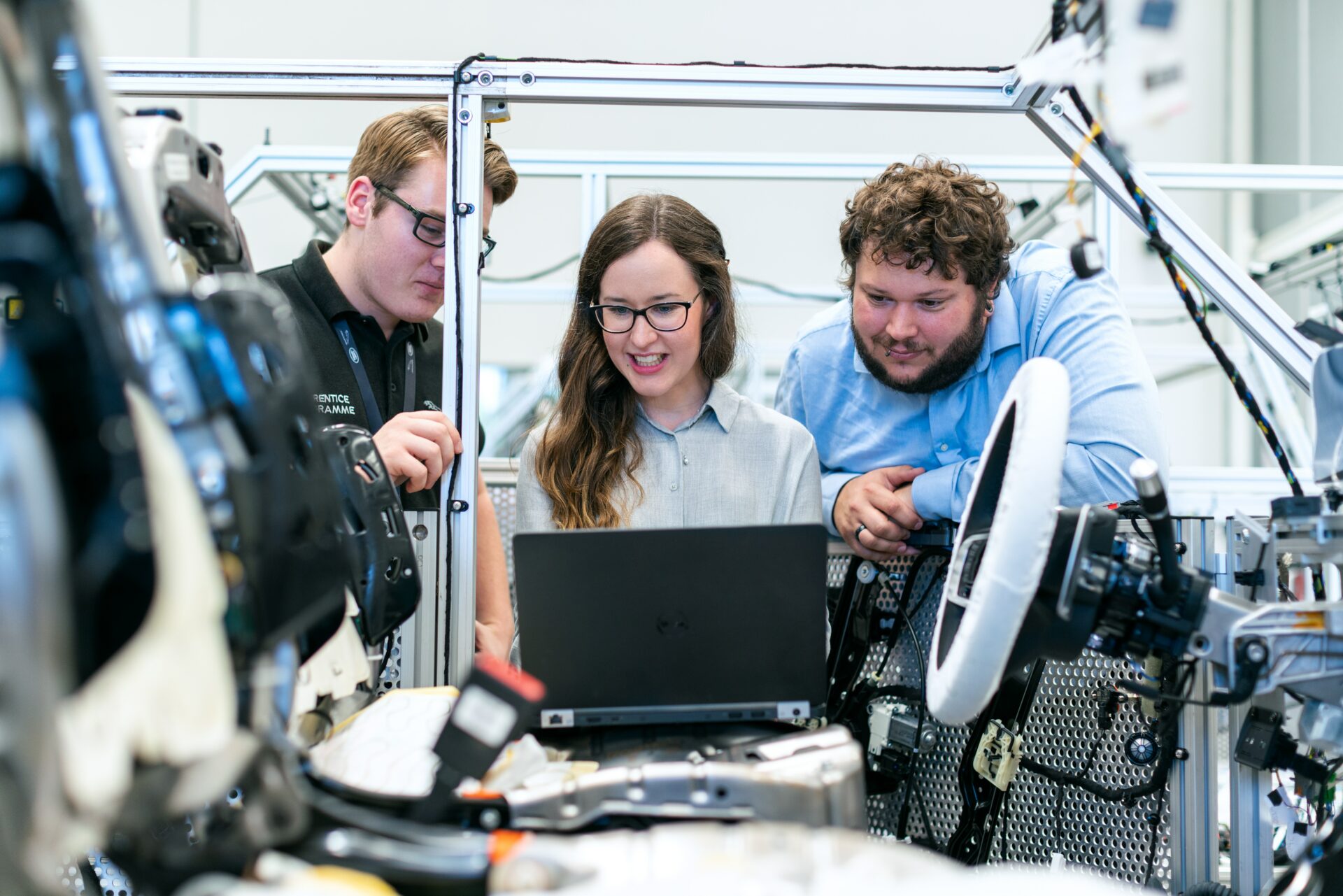
Data-Driven Industry 4.0 Applications: Empowering Industries for Educated Decisions and Continuous Improvement
Data has been recently characterized as the oil and the gold of the 21st century. In an era of rapid digital transformation of industrial enterprises, data is what empowers the optimization of business processes in many different sectors, including manufacturing, energy, oil and gas and supply chain management.
In the manufacturing space, enterprises are accelerating their digital transformation as part of the fourth industrial revolution (i.e., Industry 4.0). Industry 4.0 introduces Cyber-Physical Production Systems (CPPS) in the manufacturing shop floor, which enables the collection of large amounts of digital data about physical processes in the plant.
These data can be combined and analysed in conjunction with other datasets stemming from operators, the supply chain, and business information systems (e.g., ERP (Enterprise Resource Planning Systems)).
Based on this analysis plant operators and business management can derive useful insights on how to drive optimizations in industrial processes and to improve manufacturing decisions. Overall, Industry 4.0 facilitates data-driven manufacturing, which offers several compelling value propositions to manufacturing enterprises.
Data-Driven Manufacturing: The Benefits
Leveraging on large amounts of data and their analysis, manufacturers can enjoy the following benefits:
- Visibility on Assets, Processes and Manufacturing Performance
By collecting and processing digital data from the shop floor and the supply chain, manufacturers can instantly gain visibility on the status of assets and processes.
Furthermore, using these data, manufacturing organizations can calculate KPIs (Key Performance Indicators), which help managers take proper decisions for processes like assets’ maintenance, shifts allocation, production quality, and supply chain performance.
- Advanced Insights with Machine Learning and Artificial Intelligence
Digital data can nowadays be analysed by means of AI algorithms, such as deep learning and machine learning, towards extracting advanced insights on production processes. For example, by employing predictive analytics on machines’ and equipment data, it is possible to predict the Remaining Useful Life (RUL) of an asset towards unlocking the potential of predictive maintenance.
In another instance, the execution of deep learning analytics over products’ data (e.g., images of the product) enables the automated identification of defective products as part of quality control or product testing processes.
- Optimal Data-Driven Automation
Manufacturers leverage digital data to optimize automation. For example, digital data are used to increase the flexibility of production lines, through examining data associated with products and processes and customizing the operation of the line accordingly. Thanks to the use of digital data, this customization can be done faster, without a need for tedious configurations of Operational Technology (OT) systems.
As another example, the analysis of digital data can enable optimal and more automated decisions in terms of production schedules, production processes configurations, allocations of shifts and more.
- Digital Simulations and Digital Twins
Data-driven manufacturing enables powerful simulations and what-if analysis about production processes at the digital world. This makes it possible to experiment with complex production scenarios without having to test them in a pilot line or other physical experimentation facility.
In this direction, manufacturers can also implement Digital Twins that facilitate what-if analysis of production processes such as production scheduling, quality control and enterprise maintenance.
- Accurate Measurement and Tracking of KPIs
“To measure is to know – if you cannot measure it, you cannot improve it.” – Lord Kelvin
Unless you can measure an outcome, it is not possible to improve it. Hence, measuring and tracking KPIs is an integral element of continuous improvement and of the implementation of lean and agile manufacturing processes.
Data-driven manufacturing facilitates the tracking of KPIs about production processes, such as information about production cost, quality parameters, as well as waste and sustainability parameters. This is a foundation for improving production times, costs, and environmental performance.
- Remote Monitoring and Support Processes
Data-driven manufacturing based on Industry 4.0 systems enables the collection and analysis of data from remote.
This can save a considerable amount of effort and cost while reducing physical activities. The latter has been proven extremely beneficial following the recent COVID19 pandemic outbreak, where many machine vendors and tool builders had to reduce travel to remote locations.
This gave rise to remote maintenance and support activities based on technologies like Augmented Reality (AR) that leveraged digital data to provide remote cyber-presentations of the maintenance process to technicians.
Challenges and Barriers to Data-Driven Manufacturing
Despite the advent of Industry 4.0, the implementation of data-driven manufacturing systems is still very challenging, for several reasons, including:
- Siloed Systems and Data Fragmentation
Data-driven manufacturing hinges on the analysis of data from many different systems and data sources such as manufacturing databases, historian systems, and business information systems like ERP and MRP (Manufacturing Resource Planning) systems.
Unfortunately, most of these systems are “siloed” i.e., they do not “talk to each other”, which results in data fragmentation. Furthermore, the different data sources feature data in diverse formats that have different semantics. Therefore, manufacturing enterprises have a very hard times integrating and consolidating data from these systems.
- Lack of BigData Infrastructures
The amount of data generated in the Industry 4.0 era pushes conventional data storage systems to their limits. Manufacturers are currently in need of BigData management systems that combine and integrate conventional databases with historians and data lakes, towards managing structured (e.g., ERP data), unstructured (e.g., sensor and Internet of Things (IoT) data) and semi-structured data at scale.
In this direction, there is also a strong need for leveraging the scalability, capacity, and quality of service of cloud computing infrastructures.
- Legacy Systems
As already outlined, Industry 4.0 is empowered by CPPS systems on the shopfloor. Nevertheless, manufacturers are already using a significant number of legacy machines and equipment, which do not offer digital interfaces i.e., they cannot act as CPS systems. Hence, there is also a need for empowering data collection from legacy systems, which is not a trivial task.
- New Security Risks
The increased deployment of digital platforms on the shop floor and the flow of large amounts of digital data from system to system introduces new cybersecurity challenges. Specifically, as more CPS and IoT devices are deployed on the shopfloor, adversarial parties are provided with more opportunities for data breaches. Likewise, there is a need for securing digital data at the machine level, where there is a lack of widely accepted and tested security protocols.
Moreover, the ever-important human and organizational issues come on top of the above-listed technological concerns. In most cases, there is a need for a cultural shift towards digital manufacturing, which will facilitate manufacturing workers to understand and accept the increased use of data-driven technologies. Training, upskilling, and reskilling processes play an important role in this direction.
Upkip: Your Data-Driven Manufacturing Enabler
Acknowledging the above-listed challenges, our Upkip platform has been designed and developed to facilitate manufacturing enterprises to rapidly adopt and fully leverage the benefits of data-driven manufacturing.
It uses cutting edge IoT and Data analytics technologies to enable manufacturers to securely integrate and analyse data from a variety of diverse sources, including legacy systems and business information systems.
With Upkip previously “siloed” data are consolidated and stored in time-series databases, which can hold data for longer periods (e.g., years instead of days or weeks). Specifically, Upkip enables the collection and consolidation of data from “siloed” systems to analyse them towards deriving unique insights for manufacturing processes. In this way, Upkip empowers workers, plant managers, plant operators and the business management of a manufacturing enterprise to make educated decisions.
Upkip is not aimed at replacing existing systems: Rather it enables the integration of their data and makes them work towards delivering manufacturing intelligence.
One of the core value propositions of Upkip is that is facilitates the consolidation and association of relevant data from different sources (e.g., ERP, manual inputs, CNC machines, IoT Sensors) to help manufacturers find useful trends in the data.
Moreover, Upkip uses best practices and industry standards when contextualizing and shaping data, to facilitate their effective analysis.
Most importantly, Upkip provides “out-of-the-box” support for commonly used KPIs such as:
- Overall Equipment Effectiveness (OEE) per factory and per customer order.
- On-Time Delivery, including information per order component (items), per department, and per customer order.
- Performance of operators, including information for effectiveness coefficients, productivity, logged times (e.g., per machine, per operator, per different time series), and downtime performance.
- Machine performance, including process-specific CNC data (e.g., for drilling, milling, and cutting), as well as CNC data relating to ERP and maintenance datasets.
- Tool usage, such as instruments need per orders and tool-specific information (e.g., number of rotations).
- Customer Order performance, including for example information on cost deviations and on-time delivery.
- Scrap and waste-related information at various granularities, including for example waste per operator, per order, per machine and per tool used.
- Climate data, such as temperature, humidity, wind speed/direction and how these data impact production quality.
Manufacturers can leverage these KPIs to improve their production processes, optimize their decisions and ultimately improve production quality, cost, time, and sustainability.
Overall, with Upkip manufacturers start their Industry 4.0 and Data Driven Manufacturing journey on the right foot. It therefore certainly worth reading more about our product. You can also contact us for a demonstration or more inquiries.